TJ’s Completely Professional Guide to Building Sh*t (Software and Systems)
So you want to build some sh*t? So you want to read some random guy on the interweb? Well, you’ve come to the right place.
1. Introduction
This guide was actually meant to be called “System Design by Example”, but I’m on a sabbatical, so I figure I’m going to call it whatever the hell I want. Just be forewarned, this guide probably sucks and I likely will get distracted by other things and won’t finish it.
But hey, whoever you are, I hope you find it useful.
And all ridiculousness aside, if you find any errors, typos, better ways to express ideas, or things that I plain missed, please send them to <address here>.
2. General design process
Design phase
This is a brainstorming and estimation step. Break down the problem into different operations that must occur. 1A token needs to be validated. An id needs to be generated. The data must be transformed to HTML. Try to understand the problem, get some ideas out, and make some estimates for the things we don’t know. This step is just brainstorming - we don’t know what is good or bad yet. 2Wacky ideas occasionally work. Also, draw the designs out. It’s important because it’s much easier to see how we can vary the design by changing where operations occur.
Compare
Find any arbitrary limits on the application design, the fault tolerance, and recovery. 3For instance, calculate how many disks you’ll need. . This is just estimation, we only need to be accurate within an order of magnitude, so guessing is ok for the most part. 4For instance, what’s the peak traffic vs the average traffic? Well, just use 10x.
Network Storage CPU bandwidth iops cores latency space avg util tcp connect cache size replication Scale
So once we have a feel of the designs, we need to understand how they scale. Look at 100x and analyze the performance, cost, and scalability at avg and peak loads. 5Think big. Scale down. Remember we want to minimize the total amount of work we have to do later or at least know when we should be doing the work if our system is successful. At this point, we can start estimating the cost of maintenance and code changes.
- Profit 6Self-explanatory…I hope
3. URL Shortener
3.1. Topics covered
This is kind of the example that everyone does. It will generally be the dryest because I wrote most of this while I was gainfully working for the man and ostentiously pretending to be a professional. But we’re going to do the example anyway, just better. 7But not with blackjack and hookers…yet.
So while building this out, the major concepts we’ll be going through are:
- Encoding / address space
- Unique id generation. 8This will be a precursor to sharding, what a good/bad sharding scheme is, and eventually give us better understanding of consistent hashing.
- Caching (but not chained object cache-invalidation)
- Various digresssions as we try to scale each design. 9IOPS, random numbers, counters, encoding, and compression.
3.2. Scenario
We want to make an url shortener aka. a service that takes long urls http://thebestpageintheuniverse.net/ and turns them into something shorter.
- if a short url is given, we must return the longer url.
- assume we need to store a billion urls / month for 10 years with a 25 ms response time.
- The short url should be no longer than 10 characters long.
Come up with several systems and try to figure out:10In the next section we come up with three solutions. First, figure out how to generate a short url and convert it back to long url. Then map out all the fundamental operations and start placing these operations on various components on your drawing.
- How much will it cost to run?
- Given that the system will need to become more robust over time - how would the system be built to start vs the final state?
- Up to what point will it scale?
- How fast will it recover from a fault?
Now this is the part where you sit down and think of different ways designs - some hints are in the sidenotes.
3.3. Design
The first step in the design before we come up with anything at all is to understand what are the fundamental operations and where they are happenning on the system.
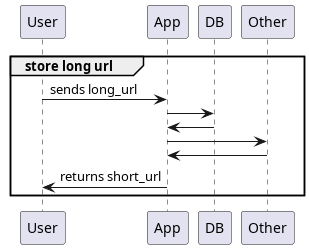
We can break the URL shortener into the following operations which each need to occur at one of the components:
- The storage and retrieval of the id and long_url
- The generation of a unique id
- The mapping/conversion of the id into a short_url that is less than 10 characters long
By changing the mapping of the operations and components, we can generate a myriad of designs which is quite handy during brainstorming sessions. If you’re not thinking of at least 2-3 ideas, how do you even know what you’re doing is any good? And it’s important to write down ideas without any judgement. I’ve utilized ideas from product managers and junior engineers more times than I can count and include one in any design meeting. There’s something to be said about diversity in thought. 11There was one time an engineer came up with a strange storage idea that everyone (including myself) analyzed and rejected. I had a nagging feeling we missed something, so that night I spent more time figuring out if it was even implementable. After some finaggling, it turns out it was. I re-presented the idea the next day and we happily rejected it because of some operations would be more complex than other solutions. Not a classic success story, but respecting people’s ideas is part of team-building. There are no bad ideas. There are just ideas that won’t work because of the conditions. Unless, of course, there are no conditions under which the idea works, in which case, it is a bad idea.
3.3.1. Operation 1 - Storage/Retrieval (rough scalability requirements)
We’re expecting a billion urls a month. Let’s make a few additional assumptions regarding the scale up and do some rounding for convenience.
- The maximum size of a URL is 512 bytes and the short url can only be 10 bytes. We can estimate this as 500 bytes / url.
- The number of reads is 10 times the number of writes.
- At some point in time, we have 100x more load on the system.
- The number of seconds in a day is 86400 which we’ll estimate as \(10^5\).
Initial estimate 12Note that depending on our storage medium, a write could take anywhere between 1 IOP and 3 IOP. We’re roughly ballparking requirements, so we don’t need a high-level of precision. See IOPS for more details.
Year | \(1\) | \(10\) |
Total urls / year | \(12 \times 10^9\) | \(12 \times 10^9\) |
Cumulative urls | \(12 \times 10^9\) | \(12 \times 10^{10}\) |
Total uncompressed storage | \(500 \times 12 \times 10^9 = 6 \times 10^{12}\) | \(60 \times 10^{12}\) |
Total compressed storage | \(3 \times 10^{12}\) | \(30 \times 10^{12}\) |
Writes / second | \(10^9 / 10^5 / 30 = 333\) | \(333\) |
Reads / second (100x writes) | \(3333\) | \(3333\) |
IOPS / second | \(4000\) | \(4000\) |
100x 13Another thing to note is that the worst-case storage requirements are 5 PB. We’ll need to calculate the cost tradeoff between disk vs cpu for compression. We’re growing at approximately 3PB / year if we compress the data.
Year | \(1\) | \(10\) |
Total urls / year | \(1.2 \times 10^{12}\) | \(1.2 \times 10^{12}\) |
Cumulative urls | \(1.2 \times 10^{12}\) | \(12 \times 10^{12}\) |
Total uncompressed storage | \(600 \times 10^{12}\) | \(6 \times 10^{15}\) |
Total compressed storage | \(300 \times 10^{12}\) | \(3 \times 10^{15}\) |
Writes / second | \(10^{11}/ 10^5 / 30 = 33 \times 10^{3}\) | \(33 \times 10^3\) |
Reads / second (100x writes) | \(333 \times 10^6\) | \(333 \times 10^6\) |
IOPS / second | \(350 \times 10^6\) | \(350 \times 10^6\) |
3.3.2. Operation 2 - Generation of the unique id
Each placement of the component generation generates a different tradeoff if we want to guarantee uniqueness.
- client-side - From a security perspective, clients can send what they want, so that means we may need to implement collision handling or use some other method to guarantee uniqueness.
- app server - This would likely be implemented as a library which is fine for single-language systems.
- database - Scaling is limited by the database if we are using the primary keys / auto-increment
- other system - An extra system specialized for id generation requires extra maintenance and we’re trading extra network latency for the ability to use the same service across many languages/systems and the ability to scale this independently. 14Not many cases where this makes sense - twitter and high-frequency trading are possibly two.
On top of that, there are a few ways to generate a unique id:
- randomness 15This could be slow or we could run out of random bits.
- monotonically increasing or random UUID’s
- hash of the URL
- hash of the URL with additional bits (time or randomness)
And in all cases, we finally need to be able to turn the id into something user-friendly enough that it can pass for a short url.
3.3.3. Operation 3 - Mapping of the id to short_url less than 10 characters long
For our system to work, we need to be able to have a bi-directional mapping from the id to a short-url and back. If we had a unique id for the long url, a simple idea encode the id into a shorter identifier. Naively, we can hash the long url to create an unique id. 16Some common hashes are MD5 or SHA which use a 128-bit address space. An md5 is of the form 7a4c751fa75da88f57e215562f9e1063 and contains 32 characters which each 16 bits. Most UUID libraries also generate 128-bit. There are shorter hashes that are more applicable to our problem.
We can use base conversion to change form of the representation. Assuming we’re using a hash like md5 our id is 128-bits and we need to figure out the appropriate encoding so the short url is less than 10 characters. 17We know base16 encoding gives length 32. A sequence of 128 bits (values of either 0 or 1) can be represented by a sequence of 32 characters between [0-9a-f] which represent \(2^4\) values. So we can group the 128 elements into groups of 4. This would be 32 groupings. In mathematical terms, \(2^{128} = (2^{4})^{32}\) A base62 encoding uses the alphanumeric characters [a-zA-Z0-9] which would satisfy the ease of use requirements. Estimating the length with a base64 representation of 128 bits would give a length of \(2^{128} = (2^6)^l\) . Taking \(log_2\) of each side, \(l = 128/6 ~= 21\). Even in base64 representation, a 128-bit id will give a length of 21 characters which is far too long for a short url and base62 would be slightly longer.
Luckily, we only need to make sure that we can store \(10^{13}\) in the worst case scenario of 100x more traffic. 18No, we don’t need a calculator.
- 10 characters of base32 gives \((2^5)^{10} = 2^{50} = (2^{10})^5 = (10^3)^5 = 10^{15}\) total urls.
- 10 characters of base64 gives \((2^6)^{10} = 2^{60} = (2^{10})^6 = (10^3)^6 = 10^{18}\) total urls.
We should also calculate the maximum address space available to us for 10 elements/characters for usability. Since \(2^{10} \approx 10^{3}\), we need a total of
\[10^{13} = 2^{10} * 2^{10} * 2^{10} * 2^{10} * 10 ~= 2^{44}\]
This means we need approximately 44 bits of address space to store all of our URLs over the lifetime of the system. These 44 bits in base32 and base64 encoding can be represented by:
- \(10^{13}\) in base32 -> \(10^{13} = 2^{44} = (2^5)^x\) . After taking \(log_2\), x ≈ 9 elements.
- \(10^{13}\) in base64 -> \(10^{13} = 2^{44} = (2^6)^x\) . After taking \(log_2\), x ≈ 8 elements.
Given that we need about 8 characters of base62 [a-zA-Z0-9] and 9 characters of base32 [a-z0-9], using base32 provides a better user experience.
Since we calculated we only need 44-bits earlier, we can use a 64-bit id. There are other hashing functions such as murmur or fnv which are fast and support lower bit-lengths.
3.4. Compare
We know the relative scale and the three major operations. We know that the storage layer likely needs to be sharded and the encoding step does not modify any state, so it can be moved and composed fairly painlessly. And, although there are more permutations, by varying where we generate the id, we can generate three major designs.19If we were talking about storing user state on the client, app, or database, we would all this a “shared nothing”, “shared something”, or “shared everything” approach and would talk about the limits of our scalability in that way.
- Database / storage layer - Generating the id on the database makes it the limiting factor, but it is straightforward to guarantee uniqueness.
- Other system - Using a 3rd party system to generate ids is an extra network hop, but the scalability limits have changed. We can have 1 id generator system per 10 app servers for instance.
App - It is simple to guarantee the uniqueness for the clients on a single app server, but we need to consider uniqueness across the system. There should be no bottlenecks here as we’re not sharing any state per client.
Now, back in the real world, it’s fairly low probability that you’ll build a system that has a billion writes per month early on. Chances are that most of your data can fit into a single database. And if you run into a big-data problem, after cleaning and sampling, you will likely end up fitting your data into memory. However, we need to understand how we can progress to be able to manage a larger system if it comes to that and not paint ourselves into a corner.
3.4.1. ID generated at the database
The simplest possible design is to use a relational database like Postgresql or Mysql to autoincrement an id and then encode it into a short url. Generally, using an RDBMs for the first phase of the application lifecycle works pretty well. 20Now, you might be thinking, well, I can use a NOSQL database like DynamoDB or Cassandra and then I can totally scale forever! But not quite. Alas, this section is the pre-cursor to sharding, but most of these databases have shards and you have a limit on the IOPS on each of these shards. For DynamoDB, you used to have a global IOP limit that is divided equally over all the shards. So, if you have a particularly hot set of keys that are pinned to a shard, your performance will be in shambles or cost you an arm and a leg. Been there, done that. Anyway, the whole point is to be able to understand the limits of the databases and understand the pitfalls rather than experiencing them first-hand.
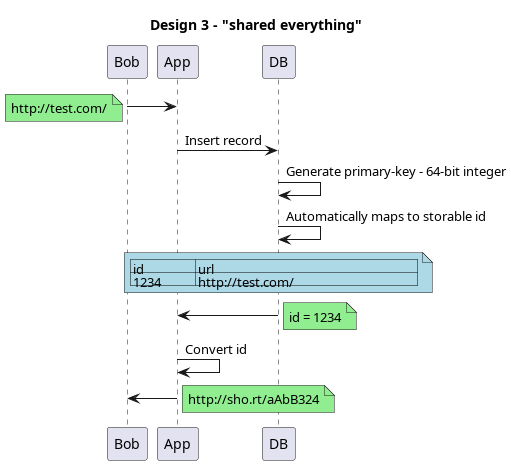
We already examined the application limits, we know the address space works fine as we need 44-bits and we can have 64-bit indexes.
But what are the other limits? When will this design fail? Can we use a single database and expect it to work? We need to examine the cpu, network, and storage, and IOPS requirements.
From the initial requirements table, we need to have a total of 50TB of storage and support a total IOPS of 4000 or 40000 at peak.
For the storage layer, if we’re using AWS, an EBS volume (gp2) can be up to 16TB with 10k IOPS per volume. And naively, we can put 23 such volumes onto a single machine.21Which, from when I tested way back when, doesn’t really work. The total storage over 10 years is approximately 60 TB. But, hopefully, having 5 such volumes would work. So from a IOPS and disk storage can be managed on a single machine.
From Aurora benchmarks, a machine can do 100k writes/s and 500k reads/s with a r3.8xlarge with 32 cores and 244 GB memory. We can probably do a smaller size and manage our peak read/write capacity of 40k. Finally, the network would be at peak \(40000 * 500 bytes = 4 x 10^4 * 500 = 2000 * 10^4 = 20 * 10^6 = 20 MB/s\) . Most systems have gbit links, so even with multiple slaves replicating, this should not be an issue.
The app server should only take a few ms to parse the user request and send it off to the database. At that point, we’re hopefully in an epoll loop 22Select, poll, and epoll are three different ways to handle IO requests in the kernel. Edge-polling (epoll) was an evolutionary step in scaling up the amount of concurrent requests can be handled. I was going to initially say file descriptors, but then my footnotes will need footnotes.. The entire request cycle should be around 10 ms, the main question is how much context-switching would affect the overall throughput. Naively, we can say each core can handle \(1 / (10 ms / request) = 100 requests/sec\) . This would mean at average we need 40 cores and at peak 400 cores. However, we know it should be much less because of the epoll loop. The application side encode/decode/http-request handling/db query should be on the order of ms. Benchmark your own code to confirm.
This covers the cpu, memory, network, and disk on the app and database. Regarding fault tolerance, we need to have a standard master-slave situation. Automated failover ranges from 30s to 90s.
So, for the initial requirements, this is the simplest design and the least amount of cost from a development and maintenance perspective.
But how would we scale this up?
Now there are a few things to know before we start, namely how all these servers connect to each other. You see, a single application server has a set of 10-20 connections it opens to the database. This is what we call a database connection pool. If you have 100 concurrent connections on the application server, each of them will time-slice the usage of the database pool. Of course, we can actually tie up the usage of the entire database connection pool with long queries, and then 80 of our clients will have to wait.
So one of the simpler ideas is to have each id generated based on a pre-selected prefix based on the database. This is essentially a pre-sharding scheme as the number of databases will need to be known beforehand. If we have 100 databases, we can prefix our generated id with the numbers 001-100 and continue using the unique id generated by the db.
Given that we need 5 PB of data, assuming that we have a maximum of 50TB of data per machine, this means we need 100 machines. This accounts for the storage volume and the overall iops, given that we don’t have any hotspots. The memory and cpu also should be approximately the same as our previous analysis.
We have to handle 400k IOPS on average and 4m IOPs at peak for reads and writes. If we can avoid hotspots, each shard will need to handle \(4 x 10^6 / 1 x 10^2 = 4 x 10^4\) . Naively, we may be able to handle peak load, but this is something that would have to be performance tested. Alternatively, we can add caches to reduce the read load, which we’ll need to handle the hotspots in any case, and keep our shard count to the minimum required for disk space.
We still need to reconsider the number of network connections and the number of app servers that we have. Given 100 shards and assuming 20 tcp connections can handle the number of requests, for each app server we would need $tcp_connection_pool_count x db_shard_count = 20 x 100 = 2000 $ . On the database end, we have app_server_count * tcp_connection_pool_count = total_connections
The next step is to understand how many app servers we need. Previously, we calculated that we need 400 cores at peak, now we would need 40k. Given 40 cores per machine, that would be 1000 app servers needed. On each database, we would have 20000 active connections for the database pool. To solve this problem, we need to add an additional layer of abstraction. This would either be a consolidated database pool between application servers or we would have to pin a set of app servers to a set of database shards or we need to move this to the client side on the request and solve this via dns.
Need a diagram describing 3 states:
- just sharded databases - how does the appserver go to the db for a particular hash?
- dns lb
- app pinning for certain urls
3.4.2. ID generated at an external system
This design requires a 3rd system to generate the ids rather than the app server or database.
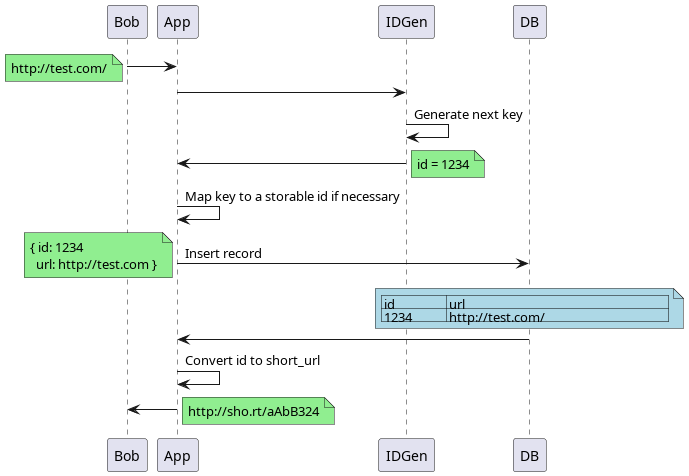
So where’s the limit on this? Likely it will be if we can increment fast enough.
In this case, we are essentially going to make a single counter. Incrementing a counter in memory takes about 0.2-0.3 microseconds given it should take 2 memory accesses and an addition. This is \(0.3 * 10^{-6} = 3 * 10^{-7}\) . This would give \(0.33 * 10^7 = 3.33 * 10^6\) increments in a second. A rough guesstimate of 3 million in a second or 3333 increments per ms. Note that this is for single threaded counter. With a multi-threaded counter, we’d have to deal with locks on the memory if we wanted accuracy or the counter to be atomic, which we need to have to guarantee uniqueness.
Although our memory is fast enough, the bottleneck occurs somewhere else. From redis benchmarks, the incr operations maxes out at 150e3 requests per second. In our first phase of the lifecycle, we only need to do 333 writes per second on average and 3333 writes per second on peak (10x).
At this point, this design requires a 3rd component, so let’s avoid the extra complexity unless necessary. We’d need to figure out how to make this resilient, which could be tricky.
3.4.3. ID generated at the app server
The real question in this design is how do we ensure that multiple application servers can generate unique ids? Because our only requirement is that we’re shortening urls and they are public, we can just use the hash of the url and encode it. But if we also wanted to offer the users a count of times it was used, we would have to make these unique, but we have a few bits to spare.
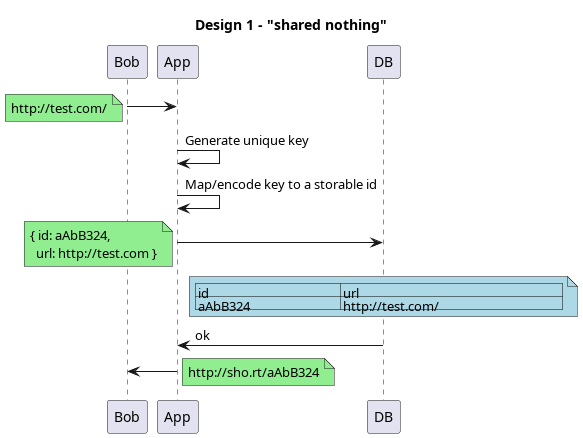
3.5. Scale the designs
Of the three main operations we are doing, in the first pass, we didn’t look in depth at the storage because in design 3 we showed that naively the data can fit on a single database server. If we have to support 100x on the storage layer, we need to store 5 PB of data. We need to understand how to shard the data, what is shared, and how to make sure there are no hotspots.
3.5.1. TODO Design 3 scaleup - pre-shard
3.5.2. Design 1 scaleup - better uuid
This was the shared nothing design where we could use a 64-bit hash. However, we can actually do better than a hash.
From the previous design, we know we need 100 shards of 50 TB. We can use the first 10-bits to represent 1024 shards, but only use the correct amount aka 100. Note that if we are using a database that represents its data as a binary tree, this will cause some write amplification on some inserts where the child pages need to be split. With a binary tree index, we are trading off random writes for easier reads. Because the hash is essentially random, the IO patterns will be mostly random, which is much slower than sequential. In fact, there are old bugs with mysql such that UUID insert performance decreases with the number of keys.
Unix-timestamp is 32-bit - which lasts from the year 1970 to 2038. Also, we can start our count from a custom epoch like 2018 instead of 1970.
We would like to include milliseconds which can be represented by 10 bits (\(2^{10} = 1024\))
64-bits total:
- 10-bits for number of shards = 2^10 = 1024 - random - we don’t want a bad hash - still can have hotspots.
- 42-bits for time in milliseconds = 70 years of time from custom epoch. We can possibly reduce the bits here since we don’t need 70 years.
- 12-bits = 2^12 = 4096 values per millisecond. We need to atomically increment a counter in some layer for this to work.
This design will allow 4k writes per ms per shard . Very conveniently, we previously calculated that we can increment a counter on a single machine around 3.5k/s. We can pre-shard to 1024 and distribute over 1 machine to start, then slowly ramp it up to n-machines as necessary.
Also, in addition to perhaps being a faster insert, another interesting property of this is that it is roughly ordered..
3.5.3. Design 2 - batching and sloppy counts
This was having a 3rd system to generate the id.
In this case, in our worst case scenario of 100x traffic x 10x peak, we’d have 333e3 requests per second.
We can still make this design work by:
- batching out groups of 10 elements and doing the rest of the counting on the app servers
- having multiple id servers - 10 servers each serving id’s of mod 10.
Also, as a side note to get faster counters, we can do sloppy counting (equivalent of batching) per CPU and try to use the L1 cache, but most applications don’t need such a hard limit.
3.6. Random ways to fail
3.7. Analysis and tradeoffs so far
We’ve analyzed three different designs so far and it appears that the dynamically generating a unique id and then inserting it appears to be the best approach for now. We have a tradeoff for the speed of inserts on the dynamically generated id which is roughly sortable vs using a hash.
Issues we haven’t tackled yet:
- sharding randomness
- how to avoid hot spots
- maintenance cost of sending modifying shards / multi-database
- TODO - DynamoDB cost vs Aurora cost
3.8. WIP - Other questions
Do we even need a cache? Calculate the depth of the btree to figure out seeks.
Branching factor of 500 (500 references to child pages, 4kB pages, depth of 4)
Size of index (number of rows vs ondisk size):
\[ 500^4 \approx (2^9)^4 = 2^{36} = 2^6 * (2^{10})^3 = 64 * 10^9 = 64 GB \]
Total storage is: \[ 500^4 * 4kB \approx (2^9)^4 * 4kB = 2^{36} * 4 * 2^{10} = 2^{48} = 2^{8} * 2^{40} = 256 * 10^{12} = 256 TB \]
- How do you know something is hot? Sloppy local counter that gets merged every x minutes + bloom filter?
- Would a trie or a hash be better for the k/v lookup for the cache? What is the space/performance tradeoff?
- Would the design also support counting the number of hits to a particular url? In this case, the number of writes > reads.
- Can we support the writes directly? If not, can we do batching? How accurate does the count need to be over what time? What is our maximum data loss?
- Introduction to CRDT - sum-only - eventually consistent.
- How would you handle expiry and the cleanup?</summary>
- At what incoming rate would the design start rejecting write requests? Read requests?
- How would you handle multi-region?
- Affinity
- Split shards across regions and do replication across even/odd shard numbers
- Write queues and read caches (hub + spoke) model - could have issues with consistency
- How do you guarantee consistency at a latency of 25 ms? What happens afterwards?
- Url compression - use earlier in example?
- Custom url - race condition - OCC?
- Spam protection?
- What is the upgrade strategy if we want to do 10^10 more urls? Can we reuse the same base encoding scheme?
- In addition, need statistics on the access of each url.
- How would the answer change if urls expired?
4. CDN
- bloom filter
- hash functions (birthday paradox) murmur/fmv/siphash
5. Appendix
5.1. Discussion of bits, bytes, and conversion
1-byte conversion (8-bits)
Let’s say in decimal we have the number 129.
Binary (base2) conversion - max value is 255 (2^8-1) 1000 0001 = 2^7 + 2^0 = 129
Hex (base16) conversion - max value is 255 (16^2 - 1) 1000 0001 = 81 8 1
129 = 8*16^1 + 1*16^0 => 81
<details> <summary>What is 129 in base36</summary> Numbers + a-z (base36) conversion 129 = 4*32^1 + 1*32^0 => 41 </details>
<details> <summary>How many single digit integers fit into a byte?</summary> ASCII values of 0-9 are 30-39 in hexadecimal. The representation of a number in ASCII needs 6-bytes, but uses a full-byte and can only represent the numbers 0-9. However, a single byte has 8-bits and can represent the values from 0-255. A 4-bit value can represent any value from 0-15, so we can actually fit two digits into a single byte.
Packed binary-coded decimal => 2 numbers can fit into 8 bits, one in the higher bits, one in the lower. Aside - BCD is generally advantageous to do digit by digit conversion to a string while binary representation saves 25% space. Can also assign different values to each bit - instead of 8/4/2/1 can have 8/4/-2/-1. </details>
8-bit clean => 7-bits for data transmission, last one for flag 7-bits of ASCII? 32 - 64 - standard ( 8-bits only, easy to copy/paste) 85 - adobe 91 - base91.sourceforge.net
5.2. IOPS - IO operations
To read and write from a disk, first it needs to “move” to the correct position and then read/write the element to the disk. The movement is known as the “seek time” and the second component is the latency which also depends on the size of the sectors and buffers.
The number of IOPS can be described by \(1 / ( seek + latency)\) . However, since \(latency = f(workload)\) and changes over different patterns it is difficult to have precision in knowing the limits of an IO subsystem. Sequential workloads have much higher throughput than random, in-place updates/writes are different than appends, etc.
An 2009 ACM report shows sequential workloads are 3x faster.
If it is a log-structured merge tree, we are trading off having fast sequential writes for random read behaviour (and later we can optimize the random read behaviour with bloom filters, hardware caches, etc) vs a binary tree which does in place updates (random io on writes instead of read).
As a rough estimate:
Device | IOPS | Interface |
---|---|---|
5,400 rpm SATA | ~15-50 IOPS[2] | SATA 3 Gbit/s |
7,200 rpm SATA | ~75-100 IOPS[2] | SATA 3 Gbit/s |
10,000 rpm SATA | ~125-150 IOPS[2] | SATA 3 Gbit/s |
10,000 rpm SAS | ~140 IOPS[2] | SAS |
15,000 rpm SAS | ~175-210 IOPS[2] | SAS |
SSD | ~10000 IOPS |
See https://en.wikipedia.org/wiki/IOPS for more details.
5.3. TODO Exponential notation
5.4. TODO Bases
5.5. Powers of Two
A quick trick to remember the powers of two is that every \(2^{10}\) multiplies by 1000.
Power | Approximation | Short |
---|---|---|
10 | 1e3 | 1KB |
16 | 65e3 | 64KB |
20 | 1e6 | 1MB |
30 | 1e9 | 1GB |
40 | 1e12 | 1TB |