Covid Policy Analysis
Detailed analysis of the risks and policies for handling the COVID19 situation based on the latest data from around the world. This document suggests policy based on scientific, moral, and fiscal viewpoints. Any resemblance of this document to the work of public health agencies is purely coincidental.
Please contact covid at sheer dot tj if you find any errors. Last updated: 2021-09-03
1. Motivation
There is always a tension between the public good and individual rights. Since the start of COVID19, governments have initiated various measures including masks, vaccines, closures, and lockdowns with the goal of reducing the total number of cases, hospitalizations, and deaths.
For many countries, there is still no end in sight. More stringent policies including vaccine passports, mandatory vaccination, and stricter lockdowns are underway to control COVID outbreaks.
What follows is an analysis of the risks and policies going forward from a scientific, moral, and fiscal standpoint based on current COVID data.
2. Policies
The present-day policies appear to be heavily politicized. This section outlines general policies that are currently in effect and compares them to an alternate strategy.
2.1. Scientific validity of current pandemic strategies and policy
Most countries have followed a strategy of vaccination and lockdown. Notable outliers to this strategy include Sweden which had a laissez-faire policy of no lockdowns and no mandatory masks. Other countries like India implemented lockdown strategies which were enforced early but were later completely ignored.
Observed policies for vaccination and lockdown in some countries include:
- Restricting access of services based on vaccination testing or status.
- Recommending or mandating vaccination for anyone older than 12 years.
- Restricting or locking down movement based on the amount of cases.
- Quarantining individuals post-travel.
Unfortunately, for the Delta variant, the premises that support these policies no longer hold true.
Falsified premises:
- Vaccinated people do not transmit the virus while asymptomatic and therefore protect the public. For the Delta variant, vaccinated and unvaccinated people transmit the virus equally for the first 5 days.1See 4.4. Viral load decreases faster in vaccinated people after 5 days, but it is unknown if that time period and viral load is meaningful with such a high r-value.
- Unvaccinated people are infected with COVID at a higher rate which means they spread the virus more. The per 100k data from Israel suggests that unvaccinated people under 70 are infected at the same rate as vaccinated people.2See 4.8.
- The vaccine is effective in reducing hospitalizations and death in all age groups. The vaccine only provides clear benefit in the 50+ age group. 3See 4.12.
- All or a majority of variants are coming from unvaccinated people. This is not falsifiable.
- Lockdowns are more effective than a laissez-faire policy in stopping the spread of the virus. Sweden provides the counter-example.
- More than 80%-90% of the population needs to be vaccinated to provide effective herd-immunity. These figures appear to discount immunity gained from catching COVID. 4See 4.13 .
Also, policies must consider:
- Social development of youth
- Risk of suicide and depression
- General economic destruction of small businesses
- Long-term needs for medical infrastructure
Policies can’t have a singular focus and be based on hope.
2.2. Neglected risk and harm reduction strategies
Every country has a slightly different policy, but the lockdown and vaccination strategy makes a huge bet on something that was never likely to be successful on its own.5I’m not sure if this is actually true, but, considering the success that Sweden has had, this strategy feels like it is worse than doing nothing. Perhaps due to the sunk cost fallacy6Humans have a tendency to continue on any course of action even though there may be a better action available. Any cost that has occurred and cannot be recovered by any means shouldn’t be a part of future decisions, but it is., some governments are doubling down on lockdowns and vaccinations rather than exploring new avenues of risk and harm reduction.
The focus on vaccination has caused us to ignore many potential harm and risk reduction strategies.
Harm reduction:
- Increase the number of outpatient treatments for COVID and non-COVID to prevent ICU overflow.
- Standardize successful treatment protocols.
- Create new ICU beds that can also serve other purposes when not in use for intensive care.
Risk reduction:
- Ensure the working population has paid sick leave.
- Provide high quality masks to the population. 7I haven’t done the analysis on this, but I would expect masks to work.
- Enhance disease monitoring to ensure detection of long-term risks such as antibody dependent enhancement, organ failure, and vaccine side-effects.
- Improve ventilation and air filtration systems in public areas.
- Create domestic medical supply chains to prevent any future issues.
- Create domestic vaccine supply chains to prevent any future issues.
- Close or monitor more tightly any BSL-3 facilities.
3. Key Findings and Policy Implications
All policies listed here are supported by the research and data analysis sections and are linked to in the side notes. Given a fixed budget, we need to allocate our resources to maximize positive outcomes. This strategy allows society to continue functioning while protecting the vulnerable population.
3.1. Vaccinations
Recommendations on vaccination for the majority of the population are not currently supported by the research.8This section is based on much research and analysis. This may change with future data, however, at the present time:
- No vaccination should be given to those under 20 years old. There are no measurable benefits in terms of infection rates9See 4.8, hospitalizations 10See 4.7, or deaths11See 4.6 and there is only unknown downside risk 12See 4.10 for this group.
- Vaccination for the 20-50 age group is likely unnecessary for healthy individuals as the risk of death is negligible. The vaccine reduces the chance of being hospitalized from roughly 1% to 0.4%.13More details at Under what conditions should someone get vaccinated? There does not appear to be any benefit in terms of protection from infection. If they exercise the precautionary principle, they will choose to remain unvaccinated.
- Vaccination for the 50+ age group or at risk individuals is recommended as it helps reduce the absolute risk of hospitalization14See 4.7 and death15See 4.6. After 2 years, most of these individuals have received a vaccination and perhaps even survived their first infection which helps their overall health outcomes. Though more investigation is necessary, additionally, the vaccine appears to reduce the amount of infections in this age group significantly. 16How effective is the vaccine at preventing infections?
- Vaccinations for anyone of any age group who previously had COVID are unnecessary. Previous asymptomatic infections may be difficult to detect, but do give immunity.17Is asymptomatic transmission relevant? Natural immunity18How effective is natural immunity? is 6x as effective as vaccination against the Delta variant even if the infection occurred over 1 year ago. If the infection occurred in February 2021, the effectiveness is 13x compared to a vaccine given at the same time. In addition, there is an unknown risk of antibody dependent enhancement that we should be cautious to avoid. 19 How harmful is the vaccine or COVID in the long-term?
- Vaccinations should not be mandated or coerced as the benefits do not warrant infringing upon individual rights. 20Are there any moral risks?
- We must stop all vaccinations if we detect antibody-dependent enhancement or if long-term harm from the vaccine begins to outweigh the benefits. 21How harmful is the vaccine or COVID in the long-term?
3.2. Mobility
- Any vaccine passports or other modes of segregation are mostly ineffective as vaccinated individuals also spread COVID at approximately the same rates during early-phase. 22Does the vaccine prevent/reduce asymptomatic transmission?
- Any quarantine procedures cannot come at any cost to the individual. This includes paid sick leave or other mandatory quarantines. 23Are there any moral risks?
3.3. Systematic changes
- Ensure production of medical supplies and vaccines is done in-country or with close allies to ensure no supply-side risk.
- Part of the vaccine budgets for the age groups that are not at risk should be re-allocated to:
- outpatient treatment plans
- building and maintaining health infrastructure
- reducing the cost of individuals to become medical professionals
- Most countries should increase the number of intensive care units (ICU) per capita to account for aging population and future risks. 24For instance, Canada can double it’s ICU capacity and operate them for 10 years with 4-8 billion dollars. Canada’s Emergency Response Benefit cost over $80B.
4. Research Analysis
4.1. How effective is natural immunity?
Several papers examining data from different time periods have shown that natural immunity is long-lasting and is more effective than being fully- vaccinated against new variants. 25I’ve had several people tell me that unvaccinated people who have had Covid have no protection which didn’t make sense at all. That’s some serious propaganda to state that the immune system stops functioning.
The most recent study 26Latest and earlier study from Israel compares 3 groups:
- vaccinated by 2021-02-28
- documented infection by 2021-02-28
- documented infection by 2021-02-28 and vaccinated by 2021-05-25 27Israel ministry allowed people to have a vaccine 3 months after an infection. Previously, this was not allowed.
They found that when they ensured the time of the original infection occurred in Jan/Feb, vaccinated people were 13x more at risk. This shows strong natural immunity for 6 months post-infection. 28Even if the infection occurred in the previous year, those who were vaccinated in Feb still had a 6x greater risk of infection. This shows stronger protection than a vaccine for at least a year.
n=16,215 each group Cases Symptomatic Hospitalization Vaccine 238 191 8 Natural immunity 19 8 1 In a 6-month period from April to November 2020, healthcare professionals in the UK (n=12,541) 29https://www.nejm.org/doi/full/10.1056/NEJMoa2034545 were tested for infection/re-infection. No vaccine was available during this time period.
n=12,541 Count Asymptomatic Symptomatic Baseline - None 11,364 100 123 Baseline - Natural immunity 1,265 2 0 - “Pilz and colleagues published a paper in European Journal of Clinical Investigation in April 2021 performing a population based study in Austria estimating re-infection rates from those infected in the first wave (Feb to Apr 2020) during the second wave (Sept to Nov 2020) vs. those not previously infected. They found 40 re-infections among 14,840 COVID-19 survivors (0.27%) and 253,581 infections in 8,885,640 of the remaining population (2.85%), showing a 91% reduced risk of re-infection.” 30Summary of Immune Protection has a bevy of additional articles.
- In addition, where the Covid variants may escape the antibodies from vaccinated individuals, the plasma from previously infected individuals could neutralize the variant under test. 31https://www.biorxiv.org/content/10.1101/2021.08.06.455491v1
4.2. Does vaccine immunity last longer than natural immunity?
From the above question, the data shows that natural immunity lasts longer and is more effective than being “fully vaccinated”. To ensure the same level of protection, additional boosters would be required that are customized to newer variants. 32I’m not sure of the long-term viability of this strategy.
4.3. Is asymptomatic transmission relevant?
We’d need to have data showing there is an immune reaction (antibody / b-cell / memory-cells) for a positive test.
The best dataset I’ve found so far is from before there was a vaccine. It examined the chance of re-infection in hospital workers.
“Of 1265 seropositive health care workers, 864 (68%) recalled having had symptoms consistent with those of coronavirus disease 2019 (Covid-19), including symptoms that preceded the widespread availability of PCR testing for SARS-CoV-2; 466 (37%) had had a previous PCR-confirmed SARS-CoV-2 infection, of which 262 were symptomatic.” 33Antibody Status and Incidence of SARS-CoV-2 Infection in Health Care Workers.
Looking at the PCR subset, only 262 / 466 had a symptomatic infection. The asymptomatic group still had sufficient antibodies to prevent re-infection compared to the baseline, however, this was with the Alpha variant. The Israel data in the natural immunity question could give us more information about the Delta variant.
4.4. Does the vaccine prevent/reduce asymptomatic transmission?
We can’t say that unvaccinated people spread the delta variant more in the first 5-7 days. We can say that vaccinated people are likely less infectious for the rest of the time period, however, we do not know if that makes a meaningful difference in the spread of COVID. 34Caveat - we don’t have any exposure baselines from any data. However, from the way exposure and load are being modeled, any selection bias doesn’t seem to be relevant.
I haven’t found any data yet to indicate the exact viral load required to transmit the virus when asymptomatic and so I’m using a threshold Ct range of 10-25 35Singapore Preprint Delta Variant Study line 288 indicates a median Ct value of 20 at the onset of symptoms. I think everyone can agree symptomatic individuals will transmit the virus. to indicate risk of transmission. This is a gross approximation but is useful for analyzing policy risk.
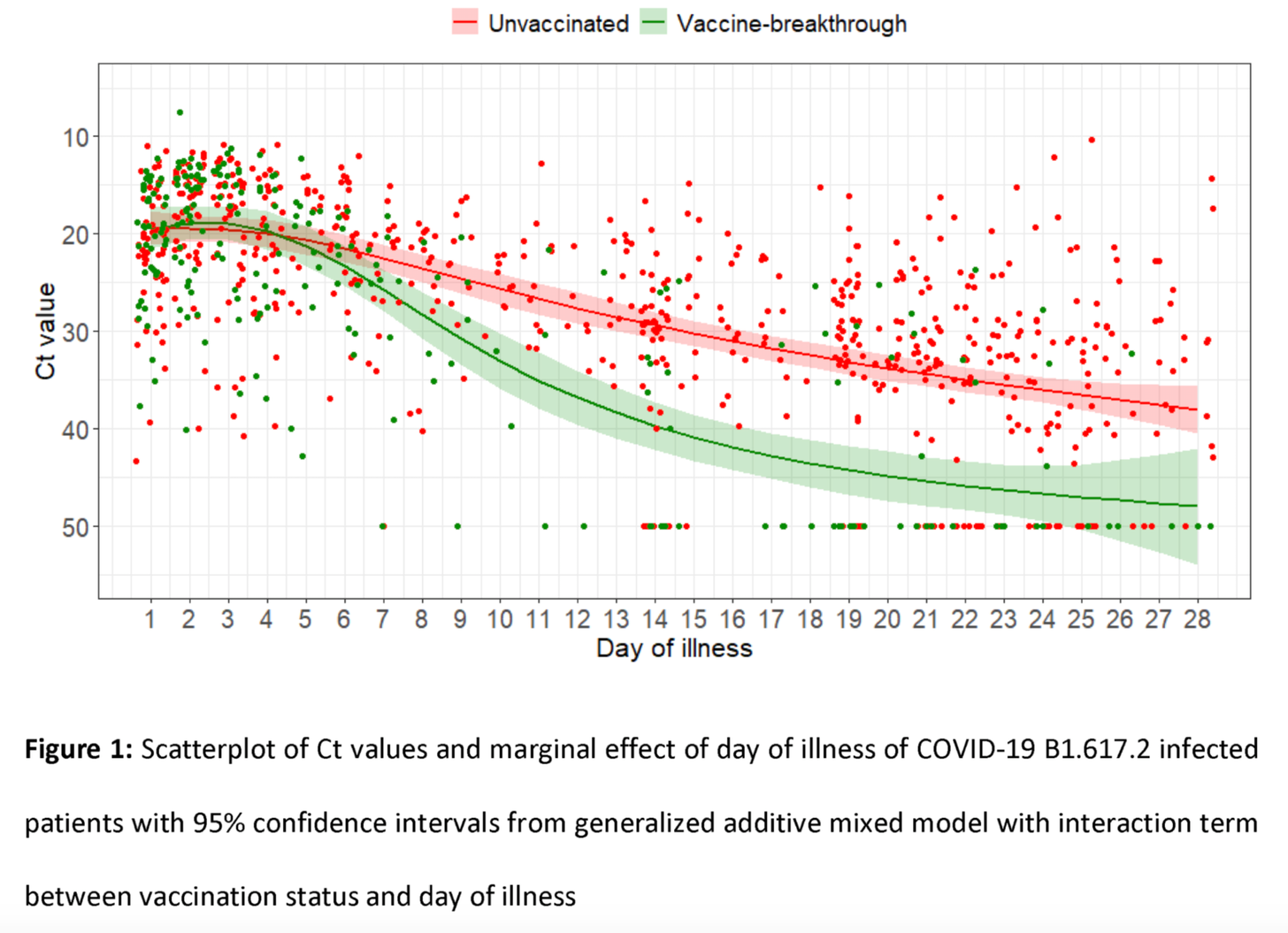
I’ve broken down the transmission into phases 36Previous study - graph at line 298. Note this data was built from a model and may not reflect real world measurements.:
Ct count | Days of illness (unvax) | Day of illness (vax) | Notes |
---|---|---|---|
10-20 | 0-5 | 0-5 | Equal load |
20-25 | 5-7 | 5-10 | Less load in vaccinated |
The CDC implemented a mask policy in the US based on the Provincetown outbreak paper 37Outbreak of SARS-Cov-2 - CDC - July, 2021 - pg 1062 which also alludes to the likelihood of equal transmission early in the infection cycle. It estimates the viral loads by using the Ct values of the diagnostic tests. The 25th to 75th percentile counts fall within 20-25(n=84) for unvaccinated people and 18-28 for vaccinated people(n=127) 38Forgive any inaccuracies, I’m reading these numbers off the graph.
Unvaccinated | Vaccinated | Total | |
---|---|---|---|
Cases | 123 (26%) | 346 (74%) | 469 |
Hospitalization | 1 (20%) | 4 (80%) | 5 |
Death | ? | ? | ? |
39The vaccination coverage of MA residents is approximately 69%.
The CDC states, “Among the vaccinated with breakthrough infections, the difficulty of detecting virus in the nasal passages, known as the cycle threshold value, was almost identical to that seen in the unvaccinated. This finding suggests that both groups carried equal viral loads and were equally likely to pass on their infections.” 40https://doi.org/10.1136/bmj.n1933
A third paper41https://doi.org/10.1101/2021.07.31.21261387 also had the same conclusion. Looking at data from June 29 to July 31, Ct values of < 25 were observed in both symptomatic and asymptomatic individuals.
Vaccinated asymptomatic | Vaccinated Symptomatic | Unvaccinated asymptomatic | Unvaccinated Symptomatic |
---|---|---|---|
9/11 (82%) | 156/225 (69%) | 7 / 24 (29%) | 158/232 (68%) |
All papers are in general agreement with common-sense modeling.42The context of the CDC paper is early transmission and the Singapore pre-print quantifies that a bit more precisely. If someone has a certain amount of viral load in their nasal passages, it certainly does make sense when breathing that they would also shed/release the virus. The amount that they shed also changes over time and is based on the severity of their infection.
4.5. Are a majority of variants coming from unvaccinated people?
This is likely unknowable.
4.6. How effective is the vaccine at preventing deaths?
The datasets analyzed tacitly assume that the death is post-hospitalization. As the virus has evolved since initial trials, we are going to look primarily at the data from the Delta variant. The data analysis is under another section.
Absolute Risk Reduction defines the amount risk is reduced when taking the vaccine: 43This notation means chance of death given someone was vaccinated vs chance of death given someone was unvaccinated.
P(death|vax)−P(death|unvax)
However, if we wanted to complicate matters, there are those who have previous immunity that could be either vaccinated or unvaccinated from a different variant. Earlier variants showed that risk of death was varying by age. The datasets could also have errors such as misattribution of cause of death44This is quite controversial. and alternate definitions of fully vaccinated. Both of these changes, would lower the mortality rate, so this analysis provides the upper-bound of the mortality rate.
The table below shows the absolute risk reduction which is commonly calculated in papers.
Age | Number needed to vaccinate | ARR |
---|---|---|
Under 50 (n=183053) | 3764 - ∞ (no effect) | 0% - 0.0272% |
Over 50 (n=21210) | 19.7 - 31.5 (24.2) | 3.1% - 5.1% |
The data shows that for those under 50, vaccination does not decrease the risk of death at all.45It is as effective as rubbing the blood from the neck of a freshly dead chicken all over your body.
4.7. How effective is the vaccine at preventing hospitalization?
To be strictly accurate, we have to exclude those with previous infections. Israel did not allow vaccinations if someone was previously infected until earlier this year, so we do have this data. For the purpose of a policy exercise, it isn’t necessary to exclude that data as this would now be the upper-bound effectiveness of the vaccine. The data classified as “severe” may be different across the sets also. 46Under 50 data is from UK data + Israel data. Under 20 data is strictly Israel.
Age | Number needed to vaccinate | ARR |
---|---|---|
UK under 50 (n=183053) | 152-219 (170) | 0.47%-0.66% |
IL under 50 (n=41115) | 131-213 (162) | 0.47%-0.76% |
IL under 20 (n=9262) | 1200-∞ (no effect) | 0% - 0.083% |
IL under 30 (n=20875) | 403-1502 (636) | 0.06%-0.25% |
UK over 50 (n=21210) | 6.4-8.1 (7.1) | 12.3%-15.6% |
IL over 50 (n=13038) | 10.7-14.7 (12.4) | 6.8%-9.3% |
47There may be some rounding errors due to significant figures in conversions.
If we are making our policy based on not overwhelming the hospital system, as most countries have, we see that for anyone over 50, there is significant reduction of risk of being hospitalized if they get infected. Under 50, the improvement is modest at best and under 20 years it is irrelevant to be vaccinated.
4.8. How effective is the vaccine at preventing infections?
It appears that this has changed drastically between the Alpha and Delta variants. 48See section 5.2 for details.
Population | Ratio with 95% confidence |
---|---|
Under 50 | 1.026 +/- 0.148 |
Under 70 | 1.063 +/- 0.114 |
Over 50 | 1.796 +/- 0.644 |
Over 70 | 2.23 +/- 0.688 |
There is no clear correlation of vaccination reducing infections for the under 70 age group. Unexpectedly, the over 70 age group shows a great improvement. More investigation and breakdown is necessary to understand what is happenning with that age group.
4.9. What are some estimates for the total number of infections reduced by vaccination?
I’m not sure I agree with the calculations, but I’m including them for completeness. This is very tricky due changes in variants and timing of vaccine rollout.
Some studies use the R-value of transmission coupled with the relative risk reduction from the vaccine to infer the reduction in infections. But we cannot attribute this improvement directly to the vaccine as natural immunity from pre-symptomatic/asymptomatic transmission is happening at the same time. The UK model estimates infection reduction by 22 million and death reduction by 60,000. 49UK Vaccine Surveillance Report Week 31
However, a better estimate might be made by looking at absolute risk reduction over time. Imagine if the vaccine was 100% effective against all variants and the R value was 10,000. Then the absolute risk reduction would be close to 100% by taking the vaccine. The higher the absolute risk, the more urgent it is to take precautions and make policy decisions. Currently, the absolute risk reduction for hospitalizations for those under 50 is 0.625%. The overall change over time may be an interesting number to calculate.
4.10. How harmful is the vaccine in the short-term?
There are various systematic reporting issues. 50Trying to figure this out really made me realize how screwed we really are in understanding anything at all.
- Vaccine side-effects are likely under-reported due to confirmation-bias. 51A friend in Africa had a child with measles. They went to the doctor and asked for treatment. The doctor asked if they were vaccinated, and since they were, they couldn’t identify the disease. When going for a second opinion, they stated the child was not vaccinated. The doctor said it was measles. Go figure. Since doctors and the public generally believe that vaccines are safe, when faced with an adverse event they may attribute it to something other than the vaccine.
- Some systems allows reporting of effects by anyone. This may cause low-quality and irrelevant data.
- Vaccine side-effects are likely to be under-reported by health professionals as the reporting is quite time-consuming.52Open letter from Dr. Hoffe in BC, CA who states that 4/900 of his patients were affected seriously. Someone sent me an interview of his and the scariest part to me was that it appeared that he found out about the side-effects almost by accident since it was a small community.
- Countries have different reporting standards. For instance, the Netherlands and France have the same amount of vaccinations given per 100 people, but reports 5x cases of harm per capita. 53The Netherlands with a population of 17M has reported substantially more cases of harm for Moderna and Pfizer (approximately 80,000) over France with a population of 65M (approximately 55,000). Cases reported from EudraVigalence up to 2021-08-28
To account for the issues in reporting, I’ve pulled only the data for serious cases for 2021-08-30. 54EudraVigalence has some subtleties to it. The fatalities could be overcounted as they apply to multiple symptoms and are only listed under grouped disorders. Due to the time required to pull all the fatality data, I spot-checked a webpage that had the data against the database to ensure it matched. The fatality data is from 2021-08-14
Vaccine | Reported by medical | Reported by all | Fatal* |
---|---|---|---|
Astrazeneca | 43107 | 187145 | 4740 |
Janssen | 4815 | 24795 | 810 |
Moderna | 34369 | 104496 | 5600 |
Pfizer | 71791 | 153145 | 10616 |
Total | 154082 | 469581 | 21766 |
To establish a lower bound of harm, there have been 526M vaccines given in the EU and 740M in Europe.55Our World in Data To establish an upper bound, we can use people vaccinated and we have 287M in EU and 400M in Europe.
1 serious case caused per | By EU vaccines | By Europe vaccines | By EU people vaccinated | By Europe people vaccinated |
---|---|---|---|---|
Serious report from medical | 3413 | 4802 | 1863 | 2597 |
Serious report from all | 1120 | 1575 | 611 | 852 |
Death | 24166 | 33998 | 13186 | 18377 |
Unless there is something terribly wrong with the EudraVigalence system or my analysis, I believe the short-term harm from the vaccine must be higher than 1/4802. From this point on, I’ll be referring going by rough approximations of magnitudes as I can think of too many sources of error.56We don’t know if the probability of a serious effect or death increases with multiple shots, so we don’t even know what denominator to use! After that, we don’t know how many cases are per person. And so on. To estimate future load on the medical system, we would actually want to know how many people obtained lifelong health issues. That is a time-consuming task left to someone other than me.57Also, due to the complexity of the VAERS system, I have not analyzed it. Many other have though.
The below table includes the upper and lower bounds as to what the chance of having a severe case or death might be. I would additionally think that this should be dependent on age, but have not analyzed the data.
Chance of | Lower bound | Upper bound |
---|---|---|
Serious case | 1/4802 = 0.021% | 1/611 = 0.163% |
Death | 1/33998 = 0.00295% | 1/13186 = 0.00758% |
4.11. How harmful is the vaccine or COVID in the long-term?
This is a known unknown which we need to account with our policies. There are a few sets that we have to look at:
- People who have not been vaccinated, but had COVID (unvax+covid)
- People who have been vaccinated, but never had COVID (vax+nocovid)
- People who have been vaccinated and had COVID (vax+covid)
For the set of people unvax+covid, we can clearly identify the set, but we have to be very careful when attributing damage in the case of using experimental treatments or drugs. For instance, there have been claims that COVID causes kidney damage in severe cases when someone was hospitalized. But how can we be absolutely be sure that this was caused by COVID rather than the treatment for COVID? Some care in necessary in disentangling the long-term effects.
For the set of people vax+nocovid we can try to find:
- Reported harm from vaccine. This would include blood diseases, long-term immune disorders, etc. The data from the previous section would need to be analyzed. Despite the lack of causation in some of these disorders, we have to use Occam’s razor and attribute any issues to the vaccine that occur with a certain timeframe or over the baseline rate. Any other position seems to be ethically challenging.
- Whether antibody-dependent enhancement will be an issue.58This paper is pretty scary. Essentially, having a vaccine can actually increase your risk of having a severe case. If this is true, that means we could see additional severe cases in the future for vaccinated groups which is measurable.
For the set of people vax+covid, we got another attribution problem.
We would have to examine
- additional excess case load.
- organ transplant lists
- etc.
These are the types of things we need to try to understand to avoid overwhelming the medical system in the long-term. If any of this section has been measured, I haven’t seen it yet.
From a policy perspective, vaccinations should be stopped under the following conditions:
- we detect antibody dependent enhancement.
- we measure the long-term diseases caused by vaccination to be higher than “something.” “Something” could be the death rate of COVID, unvax+hospitalizations, etc, etc.
Also, if we err on the side of caution and with all other things being equal, we would have to not recommend vaccinations due to
- the conditional probability of having to catch COVID first
- and a person may get COVID related disorders while vaccinated or unvaccinated.
If someone makes a choice to vaccinate, they are definitely in the vaccine group. If someone chooses to not vaccinate, they may not enter the unvax+covid group for a long time.
4.12. Under what conditions should a person get vaccinated?
We’ve seen that from earlier sections that we are primarily trying to make the first infection as harmless as possible.
Considering that vaccination status does not protect other members of the public, we are looking for59We want the harm from covid when being unvaccinated to be greater than the harm of covid when vaccinated and the harm of getting the vaccine.:
P(harmcovid|unvax)−P(harmcovid|vax)>P(harmvaccine)
On the left hand side, we are considering harm to be a serious illness resulting in hospitalization, ICU, or death by age group.
On the right hand side, we have to categorize the vaccine harm as short term which are detected by immediate reports and the long-term which is harder to quantify. We are going to ignore the known-unknown risks from COVID and the vaccine, but err on the side of caution based on the reasoning in the previous section.
The below table shows a decision matrix with known and unknown risks with several major caveats.
- The baseline risk of catching COVID is not known.
- The improvements from the vaccine are given by risk reduction which requires catching COVID while the harm from vaccine does not.
- The rate of infection for vaccinated people vs unvaccinated people is roughly equal under 50 and much higher for unvaccinated people over 50. 60See section 5.2 .
From 4.10, we have the following table:
Chance of | Lower bound | Upper bound |
---|---|---|
Serious case | 0.021% | 0.163% |
Death | 0.00295% | 0.00758% |
Given you take the vaccine:
Age | ARR of death from COVID | Risk of death from COVID | ARR of severe COVID | Risk of severe COVID | Should vaccinate? |
---|---|---|---|---|---|
<20 | No data | No data | No significance | 0.034% | No |
20-50 | 0.0%-0.0265% | 0.0285% | 0.47%-0.76% | 0.68%-1.4% | Up to you |
50+ | 3.1%-5.1% | 5.6% | 6.8%-15.6% | 11%-18% | Likely |
The 20-50 age group61The under 20 age group data is from Israel. There was no statistical difference over n=8000 in reducing the risk of severe effects. The UK dataset provides no significance over n=183053 for risk of death under 50. will need to be given the data and make their own decision. From a societal perspective, we need to have a roughly equal split among vaccinated and unvaccinated in that age-group to buffer society against a bad outcome for either of the groups.
The chances of survival is 99.97%. Although I’ve put the confidence intervals for the risk reduction, there is no significance, or in other words, we have no idea if it helps or not. A person has the same risk of dying(0.03%) in a motor vehicle accident in some US states. 62Car accident deaths are not the correct statistical comparison, but I’ve spent 150 hours writing this and am tired of being precise.
The risk from a hospitalization from COVID, given a 100% chance of getting the disease, is roughly 1%. Taking the vaccine, could reduce it by 0.6%. A healthy 40 year old could improve their odds from 99% to 99.6% and take on a slight risk of side effect of 0.16%. Whether this is enough of a benefit to take on a different risk, well, that is up to the person. If the group follows the precautionary principle, they will likely skip the vaccination as both the risk and benefit are too low.63There are mild cases of COVID. There are no mild cases of Ebola.
4.13. How much of the population needs to be vaccinated to achieve herd immunity?
This is another very tricky question. Some of the factors that herd immunity depends on are:
- the effectiveness in reducing infections by vaccination or natural immunity
- the timeframe the immunity is effective.
In the UK, blood donations have shown that64Vaccine Surveillance Report Week 31 (UK):
- 16% have antibodies that came from infection
- 96% have antibodies that came from both vaccination and infection.
There is likely a large overlap in the set of people who donate blood and who vaccinate, so the overall distribution may differ quite a bit.
The 16% who have antibodies from infection will have much longer lasting immunity than those that are vaccinated.
Unfortunately, I’m not sure how to answer this question more precisely at this time. Any claims that a 80%-100% vaccination rate is required to achieve herd immunity is likely incorrect as it discounts those who now have natural immunity without being vaccinated.
4.14. Are there any moral risks?
A moral based policy approach requires a standard that any policy will not cross. Born out of World War II, the Nuremburg Code 65We can adapt it from medical experiments to be generally true. provides a basis for this structure and states that any medical procedure requires informed consent.
“The voluntary consent of the human subject is absolutely essential. This means that the person involved should have legal capacity to give consent; should be so situated as to be able to exercise free power of choice, without the intervention of any element of force, fraud, deceit, duress, overreaching, or other ulterior form of constraint or coercion66Financial coercion is the most common form today.; and should have sufficient knowledge and comprehension of the elements of the subject matter involved as to enable him to make an understanding and enlightened decision.”
This means:
- There can be no policy that requires vaccinations without consent.
- No coercion, financial or otherwise, may be used to influence vaccination choice.
Even if it is for great public benefit, 67When I was in my 20’s, I believed the ends justify the means. Sometime in my 30’s, I decided I preferred to sleep well instead. we shouldn’t do things that would be considered war crimes 50 years ago.
Practically, a person convinced against their will is of the same opinion still. Violating these two points will likely cause large scale protests and a segregation of society.
5. Data Analysis
5.1. Hospitalizations and Deaths
5.1.1. Flu Absolute Risk Reduction (ARR) / NNT Baseline
The Number Needed to Treat (NNT) is a measure of how effective a treatment is. 68https://en.wikipedia.org/wiki/Number_needed_to_treat
It is the average number of patients who need to be treated to prevent one additional bad outcome (e.g. the number of patients that need to be treated for one of them to benefit compared with a control in a clinical trial). It is defined as the inverse of the absolute risk reduction.
The ideal NNT is 1 and the NNT for antibiotic treatments for sinusitis is 1.5 69https://pubmed.ncbi.nlm.nih.gov/18425861/ . In comparison, the NNT for flu vaccine is 29 (0.0344 ARR) for minor illness and 71 (0.0141 ARR) for influenza. This is stated to have a “very modest effect.” 70https://doi.org/10.1002/14651858.cd001269.pub6 and https://doi.org/10.1002/14651858.cd001269.pub5
5.1.2. England
Looking at the delta variant shows interesting results in a breakdown by age. 71SARS-CoV-2 variants of concern and variants under investigation in England (July 23 2021). Referenced data comes from pg. 18 table 5.
Age: < 50 | Unvaccinated | Two doses | Any doses |
---|---|---|---|
Cases | 119063 | 15346 | 63990 |
Hospitalization | 1712 | 140 | 564 |
Deaths | 34 | 4 | 10 |
% Hospitalized | 1.44 | 0.91 | 0.88 |
% Dead of hospitalized | 1.99 | 2.86 | 1.77 |
The data breakdown shows that it is almost irrelevant to be vaccinated if you’re under 50. Roughly 1% of people who got Covid were hospitalized, of those people roughly 2% died. That is a survival rate of roughly 99.98%. Note that the number of deaths is so low, that any variability throws intuition out of whack. For instance, if there were no deaths in the two dose group at this sample size, would we say that there is no chance of death after hospitalization? 72It was a rhetorical question, the answer is no. Currently, the 4 deaths in the two dose groups gives a higher probability of death than being unvaccinated. It could be possible that the death rate is roughly equal across all cohorts once they are hospitalized as Covid causes complications from existing issues. The data below reflects that hypothesis, but it can certainly be disproved with more data. 73The absolute risk reduction of death is (34/119063 - 10/63990 ) = 0.013% which translates to a number needed to treat of approximately 7700. If we use just the two doses data, this number is infinity. Either way, this means the vaccine is fairly ineffective for this cohort in preventing serious disease or death. To prevent severe cases, it is 1712/119063 - 564/63990 = 5.56e-3 which is an NNT of 179.7
Age: >= 50 | Unvaccinated | Two doses | Any doses |
---|---|---|---|
Cases | 2337 | 13427 | 18873 |
Hospitalization | 440 | 703 | 912 |
Deaths | 131 | 220 | 279 |
% Hospitalized | 18.83 | 5.24 | 4.83 |
% Dead of hospitalized | 29.77 | 31.29 | 30.59 |
The data for those above 50 shows it is important to have a single shot. Only 5% of people who had a shot were hospitalized while it is roughly 20% for the unvaccinated. As per the previous table, the percentage of death once hospitalized remains constant and much higher than the under 50 group at 30% vs 2%. 74The absolute risk reduction of death is (131/2337 - 271/18873 ) = 4% which translates to a number needed to treat of ~ 24 which isn’t great, but not completely ineffective. The absolute risk reduction in hospitalization is (440/2337 - 912/18873) ~ 0.139 and corresponds to an NNT of 7.1 which is better than the flu vaccine.
5.1.3. US Los Angeles
Going through CDC - Morbidity and Mortality Weekly Report Aug 24, 2021 a claim is made that the hospitalization rate of unvaccinated was 29 times that of vaccinated based on a rolling75Quite frankly, I just don’t understand why anyone would look at a 7-day incident rate, let alone have the media headline it. There are many ways for the data to be skewed (length of stay, quicker death is better for this statistic, etc.) 7-day incident rate. If we look at the totals from the table, we would say the hospitalization rate is roughly 2x for unvaccinated (7.6% vs 3.2%).
Los Angeles | Unvaccinated | Fully vaccinated | Any doses |
---|---|---|---|
Cases | 30801 | 10895 | 12326 |
Hospitalization | 2355 | 350 | 439 |
Deaths | 176 | 24 | 31 |
% Hospitalized | 7.65 | 3.21 | 3.56 |
% Dead of hospitalized | 7.47 | 6.86 | 7.06 |
This dataset does not have the breakdown of hospitalization or deaths by age. As it is, we can see that the rate of death once hospitalized is constant over all the groups which agrees with other data sets. A hypothesis from this data is that with a breakdown by age the same behaviour for % hospitalized as the previous data sets would occur with the risk to under 50 being negligible and the risk above 50 being substantial. 76Absolute risk reduction of 2355/30801 - 439/12326 = 0.0408 making an NNT of 24.4 for hospitalization. Absolute risk reduction for death is 176/30801 - 31/12326 ) = 3.2e-3 which corresponds to an NNT of 312.5
One interesting thing to note is the breakdown by race. There is not enough data to come to any conclusion, but first-generation immigrant families are sometimes large and multi-generational households with lower socio-economic status, so it would be interesting to see the overall risk profile of the elders in those households.
5.1.4. Israel
The Israel raw data provides normalized numbers, but it does not appear to have death rates. As we have been using non-normalized numbers and the normalized numbers do not seem to indicate different policies, to remain consistent the non-normalized numbers are used here. The normalized table and raw data is provided in the appendix.
Age: < 50 | Unvaccinated | 2-dose | Any doses |
---|---|---|---|
Cases | 12886 | 26879 | 28229 |
Severe | 88 | 17 | 19 |
Deaths | ? | ? | ? |
% Severe | 0.68 | 0.06 | 0.07 |
% Dead of severe | ? | ? | ? |
Age >= 50 | Unvaccinated | 2-dose | Any doses |
---|---|---|---|
Cases | 2379 | 10469 | 10659 |
Severe | 262 | 298 | 312 |
Deaths | ? | ? | ? |
% Severe | 11.01 | 2.85 | 2.93 |
% Dead of severe | ? | ? | ? |
For the under 50 cohort, we see that the risk of severe disease is < 1%. Unlike the UK dataset, the differences are significant between vaccinated and unvaccinated using a two-sided hypothesis. We can be pretty sure this isn’t random chance. 77Absolute risk reduction is (88/12886 - 19/28229) = 0.006156 corresponding to an NNT of 162. This is much worse than the flu vaccine in preventing severe disease.
For the over 50 cohort, we can see a significant improvement when vaccinated. This is consistent with the UK data set. 78Absolute risk reduction is (262/2379 - 312/10659) = 0.00435 which gives an NNT of 12.4.
5.1.5. Summary
The UK and US data sets use hospitalization and death in their data set. Israel uses “severe” which we are taking to mean roughly the same. The ARR is calculated from previous sections and turned into an NNT with confidence interval to make it more clear. 79I didn’t feel like re-deriving the formula for the 95 percentile confidence interval: SE(ARR)=1.96∗√((pa∗(1−pa))/ta+(pb∗(1−pb))/tb)
Data/cohort | NNT (severe) | NNT (death) |
---|---|---|
UK < 50 (n=183053) | 152-219 (170) | 3764 - ∞ (no significance) |
Israel < 50 (n=41115) | 131-213 (162) | ? |
UK >= 50 (n=21210) | 6.4-8.1 (7.1) | 19.7 - 31.5 (24.2) |
Israel >= 50 (n=13038) | 10.7-14.7 (12.4) | ? |
US Los Angeles | 22.4-27.9 (24.9) | 226.2 - 505.5 (312.6) |
An interesting thing to note is that 30% of all individuals that are over 50 die if they are hospitalized regardless of vaccination status from the UK data. The vaccination prevents hospitalization for that age group. The US LA data isn’t so useful as it isn’t segregated by age.
5.2. Infection of unvaccinated vs vaccinated
This is based on the data obtained from https://datadashboard.health.gov.il/COVID-19/general and raw data is in the appendix.
age group | cases unvax/ per 100K | cases vax/100K | cases part vax/ 100k | total cases vax/100k | Ratio of unvax / vax cases |
---|---|---|---|---|---|
“12-15” | 1230.8 | 135.2 | 884.5 | 1019.7 | 1.2070217 |
“16-19” | 1248.2 | 568.6 | 507.8 | 1076.4 | 1.1596061 |
“20-29” | 1119.1 | 848.4 | 580 | 1428.4 | 0.78346402 |
“30-39” | 1355.4 | 857 | 526.7 | 1383.7 | 0.97954759 |
“40-49” | 1210.3 | 790.6 | 408.4 | 1199. | 1.0094245 |
“50-59” | 1246.4 | 660.5 | 478.1 | 1138.6 | 1.0946777 |
“60-69” | 1039.8 | 404.9 | 455.5 | 860.4 | 1.2085077 |
“70-79” | 2008.9 | 331.7 | 353.7 | 685.4 | 2.9309892 |
“80-89” | 1677.1 | 460.2 | 452.8 | 913. | 1.8369113 |
“90+” | 2545.2 | 642.1 | 670.8 | 1312.9 | 1.9386092 |
The following table summarizes the result:
Population | Ratio with 95% confidence |
---|---|
Under 50 | 1.026 +/- 0.148 |
Under 70 | 1.063 +/- 0.114 |
Over 50 | 1.796 +/- 0.644 |
Over 70 | 2.23 +/- 0.688 |
The result is interesting and somewhat unexpected. I would have expected that the ratio for infection rate between age groups to be relatively consistent. This may be explained by Israel starting a 3rd round of shots for the at-risk / over-50 population.
- For the under 50 age group, vaccination is not correlated with the rate of getting COVID.
- For the over 50 age group, vaccination is correlated with reducing the chances of getting COVID significantly.80The large error bars on confidence interval are due to the lower rates from 50-70. This could be due to boosters, socioeconomic indicators, co-morbidities, etc.
More investigation is certainly necessary, but from a policy perspective we have sufficient data.
6. Conclusion
Many policies for the vaccination and lockdown strategy are no longer supported by the current data for the Delta variant. 81And please let me know if I made an error.
Multiple premises supporting these policies were falsified in Section 2.1:
- Vaccinated people do not transmit the virus while asymptomatic and therefore protect the public. For the Delta variant, vaccinated and unvaccinated people transmit the virus equally for the first 5 days.82See 4.4. Viral load decreases faster in vaccinated people after 5 days, but it is unknown if that time period and viral load is meaningful with such a high r-value.
- Unvaccinated people are infected with COVID at a higher rate which means they spread the virus more. The per 100k data from Israel suggests that unvaccinated people under 70 are infected at the same rate as vaccinated people.83See 4.8.
- The vaccine is effective in reducing hospitalizations and death in all age groups. The vaccine only provides clear benefit in the 50+ age group. 84See 4.12.
- All or a majority of variants are coming from unvaccinated people. This is not falsifiable.
- Lockdowns are more effective than a laissez-faire policy in stopping the spread of the virus. Sweden provides the counter-example.
- More than 80%-90% of the population needs to be vaccinated to provide effective herd-immunity. These figures appear to discount immunity gained from catching COVID. 85See 4.13 .
This document has shown where vaccination is beneficial and where it is not. To summarize, it appears to be effective for those over 50 or with health issues, but of limited benefit for all other age groups. From the section on key findings:
- No vaccination should be given to those under 20 years old. There are no measurable benefits in terms of infection rates86See 4.8, hospitalizations 87See 4.7, or deaths88See 4.6 and there is only unknown downside risk 89See 4.10 for this group.
- Vaccination for the 20-50 age group is likely unnecessary for healthy individuals as the risk of death is negligible. The vaccine reduces the chance of being hospitalized from roughly 1% to 0.4%.90More details at Under what conditions should someone get vaccinated? There does not appear to be any benefit in terms of protection from infection. If they exercise the precautionary principle, they will choose to remain unvaccinated.
- Vaccination for the 50+ age group or at risk individuals is recommended as it helps reduce the absolute risk of hospitalization91See 4.7, death92See 4.6, and infection93How effective is the vaccine at preventing infections?.
- Vaccinations for anyone of any age group who previously had COVID are unnecessary. Previous asymptomatic infections may be difficult to detect, but do give immunity.94Is asymptomatic transmission relevant? Natural immunity95How effective is natural immunity? is 6x as effective as vaccination against the Delta variant even if the infection occurred over 1 year ago. In addition, there is an unknown risk of antibody dependent enhancement that we should be cautious to avoid. 96 How harmful is the vaccine or COVID in the long-term?
- Vaccinations should not be mandated or coerced as the benefits do not warrant infringing upon individual rights. 97Are there any moral risks?
- We must stop all vaccinations if we detect antibody-dependent enhancement or if long-term harm from the vaccine begins to outweigh the benefits. 98How harmful is the vaccine or COVID in the long-term?
Also, there is little reason to limit or punish those that choose not to get vaccinated with passports and tests.
- Any vaccine passports and other modes of segregation are mostly ineffective as vaccinated individuals also spread COVID at approximately the same rates during early-phase. 99Does the vaccine prevent/reduce asymptomatic transmission?
- Any quarantine procedures cannot come at any cost to the individual. This includes paid sick leave or other mandatory quarantines. 100Are there any moral risks?
Many countries are over a year into a strategy of vaccination and lockdown. This has not brought us back to a functioning society.
The data shows the vaccine has worked well for those over 50 or with health conditions, but has hardly helped in any other areas.
This issue is becoming increasingly political. We need to put that behind us, publish all the raw data, and justify our policies going forward.
7. FAQ
7.1. Why should I trust you over an expert or an authority?
As an ex-physicist and semi-retired software architect I’m well-versed in analysis. I’m not in the medical profession or a politician, so I don’t have any of the inherent biases from those fields. I consulted with experts who were both pro-vaccine and anti-vaccine and now know far more than I ever wanted to about COVID. As the conclusions from both sides were tinged with emotion, I resorted to finding datasets that could show conclusions.
This is not about trusting a person or intuition, this is about looking at the data rationally. Experts have been wrong in the past and will be wrong in the future and appeal to authority is a poor argument when you can dive into the data.
This document has taken over 120 hours to create as of 2021-09-03 and I have not being compensated in any way. I have gotten feedback from various professors, current and ex-researchers at pharmaceutical companies, doctors and nurses, ex-physicists, and extremely technical people in the software and chip industries. I have offered to include their names on the document due to their extensive input, however, due to the political nature of the topic they preferred to remain anonymous.
Regardless of trust level, I suggest you read the appendix which contains Sagan’s how to detect baloney and how to account for common biases.
7.2. You are wrong. Your analysis is flawed by X.
That is certainly possible. In addition, the virus evolves and so data will change and policies should be based on latest and most comprehensive datasets. This document is accurate as of 2021-08-28. If you have better or more accurate data, please send it to me and if I have made an error in analysis, I would absolutely love to know where.
In addition, the analysis can be improved in the following ways:
- Author bias. Although I try to be un-biased, that is impossible. 101I think that people who agree with me are generally more intelligent.
- Only basic arithmetic is used.
- Publicly available data sets from papers are used sometimes instead of raw data.
- More comprehensive breakdowns would involve existing mobidity, previous infection, re-infection, immunocompromised, percentage of 1-shot who also had COVID, etc. None of that has been taken into account.
- Each country certainly does measurements differently. No normalization was taken into account and likely isn’t possible.
- All economic numbers are gross estimates which may be off by an order of magnitude.
- So far policies are based on cases, hospitalization, and death. This analysis has not taken into account non-death issues caused by COVID.
- Examining % dead of hospitalization may not account for all deaths. Unfortunately, the data sets that I’m using don’t appear to account for this either.
- Using unnormalized rates could show vaccines are either slightly better or worse than they are based on the percentage vaccinated especially on smaller data sets. Normalized data was only provided by Israel and did not change the overall results meaningfully in terms of policy.
- The VAERS data was not examined and the Eudra data should be examined more throughly. There is likely also an age correlation here.
7.3. Why should I trust the data you’re using?
I’m using published data sets from government sources and studies. The majority of the conclusions are drawn from:
- Israel Data Dashboard which provides raw data
- UK Bi-weekly reports
- CDC
- EudraVigalence
- Various studies
Unfortunately, raw data sets outside of Israel are very hard to come by, so it takes quite a bit of digging to be able to come to any conclusions. If you know of any better data sources, please let me know. I’m looking for cases by age, vaccine status, vaccine type, co-morbidities, mild/severe/death, etc.
Also, all data used was pulled from sources in 2021-08. In the future when boosters are available in general, it could be the case that institutions redefine the meaning of fully vaccinated. As long as those without boosters are defined as partially vaccinated, this document will be consistent. If those without boosters are defined as unvaccinated, well, that would be a big problem for data worldwide.
7.4. You are clearly a pro-vaxer.
Sorry, I can’t change the data. I’ve tried to present a completely unbiased view. Please read bias section and scientific analysis section. If the data changes, I’ll change my opinion.
If you have data or analysis that gives a different viewpoint on policy, please let me know so that it can be analyzed.
7.5. You are clearly an anti-vaxer.
Sorry, I can’t change the data. I’ve tried to present a completely unbiased view. Please read bias section and scientific analysis section. If the data changes, I’ll change my opinion.
If you have data or analysis that gives a different viewpoint on policy, please let me know so that it can be analyzed.
7.6. But look at this youtube video!
No.
7.7. But look at graph Y published by Z.
I’m looking for data that is:
- Broken down by age groups.
- Preferably normalized per 100k and with unnormalized counts.
- Preferably with secondary tables for co-morbidities.
If it has at least one of these, please send it to me!
But without data broken down by age group, it doesn’t tell us very much.
7.8. But the vaccine is 90% more efficient! Everyone says so!
That is using relative rates.
Because the raw numbers we are dealing with are so small, any miniscule changes in percentage calculations can easily cause them to be meaningless. For instance, in the UK data set for under 50, there are a total of 34 deaths/119063 cases for unvaccinated, and 4/15346 for 2 dose vaccinated.
We could technically calculate the efficiency as: 100∗(1−vaccinated/unvaccinated)
At the numbers listed above, we would have 93% efficency. However, if there is just one more death in the 2-dose vaccinated group, we suddenly have -14% efficiency.
The calculations for Absolute Risk Reduction (ARR) and Relative Risk Reduction (RRR) are basic arithmetic, however, even the fact-checkers need to be fact-checked. The Reuters fact check from June 2, 2021 of social media posts critiquing a Lancet paper is technically accurate. The Lancet paper is also technically accurate. 102And very importantly stated that the absolute risk reduction depends on the background risk which depends on the conditional probability of being infected.
FDA recommends, “Provide absolute risks, not just relative risks. Patients are unduly influenced when risk information is presented using a relative risk approach; this can result in suboptimal decisions. Thus, an absolute risk format should be used.” 103https://www.ncbi.nlm.nih.gov/pmc/articles/PMC7996517/pdf/medicina-57-00199.pdf
Scientists can be technically correct, yet still misleading or wrong due to misapplied context or technique.
7.9. But the vaccine has microchips in it! Everyone says so!
- A 5G chip would be roughly 1 cm and won’t fit inside a needle. But let us say it is indeed chip or RFID tag that can fit through the point of a needle…
- It would still need to be powered. But let us say it has a micro-turbine which can generate up to a micro-watt…
- It would still need to emit a signal that is detectable for you to be monitored through layers of conductive fluid that attenuates the signal. The amount of power required to pass a signal through 1cm of blood and muscle is much, much, much, much more than a 1 microwatt.
7.10. How do I contact you?
I’ll be periodically checking covid at sheer dot tj.
8. Revisions
- 2021-09-17 - Added additional paper for 4.4.
- 2021-09-13 - Removed unnecessary paragraphs in #vaccination_death
- 2021-09-05 - Edited section 7.1
- 2021-09-03 - First public version.
- 2021-09-03 - 5th draft. Adding conclusion.
- 2021-09-02 - 4th draft. Rewriting sections for clarity.
- 2021-09-01 - Added graphs and fixed formatting.
- 2021-08-30 - Added harm data from Eudra
- 2021-08-29 - 3rd draft
- 2021-08-27 - 2nd draft
- 2021-08-26 - Initial draft.
9. Appendix
9.1. The Fine Art of Baloney Detection
I have shortened and paraphrased an excerpt from Carl Sagan. 104Carl Sagan - The Fine Art of Baloney Detection
- Wherever possible there must be independent confirmation of the “facts.”
- Encourage substantive debate on the evidence by knowledgeable proponents of all points of view.
- Arguments from authority carry little weight — “authorities” have made mistakes in the past. They will do so again in the future.
- Spin more than one hypothesis. If there’s something to be explained, think of all the different ways in which it could be explained and how it can be disproved.
- Try not to get overly attached to a hypothesis just because it’s yours. It’s only a way station in the pursuit of knowledge. Ask yourself why you like the idea. Compare it fairly with the alternatives. See if you can find reasons for rejecting it. If you don’t, others will.
- Quantify. If whatever it is you’re explaining has some measure, some numerical quantity attached to it, you’ll be much better able to discriminate among competing hypotheses. What is vague and qualitative is open to many explanations. Of course there are truths to be sought in the many qualitative issues we are obliged to confront, but finding them is more challenging.
- If there’s a chain of argument, every link in the chain must work (including the premise) — not just most of them.
- Occam’s Razor. This convenient rule-of-thumb urges us when faced with two hypotheses that explain the data equally well to choose the simpler.
- Always ask whether the hypothesis can be, at least in principle, falsified. Propositions that are untestable, unfalsifiable are not worth much.
9.2. Different Types of Biases
- Bias from over-influence from authority. The Milgram experiment comes to mind here, but more practically, there’s a large subset of the population that blindly listens to scientists.
- Bias from dislike and hate. And to top it all off, there’s another large subset of the population that will not listen to anything scientific. 10525 years ago, I could not have possibly imagined writing that line.
- Incentive bias. Since the emergence of COVID, reporting on scientific analysis has been likely incentive-biased.106From a Charlie Munger speech - Here, my early experience was a doctor who sent bushel baskets full of normal gallbladders down to the pathology lab. [The doctor was removed from his position.] And one of the old doctors who participated in the removal was also a family friend, and I asked him, I said , “Tell me, did he think, here’s a way for me to exercise my talents,” this guy was very skilled technically, “And make a high living by doing a few maimings and murders every year, along with some frauds?” And he said, “Hell no, Charlie. He thought that the gallbladder was the source of all medical evil, and if you really love your patients, you couldn’t get that organ out rapidly enough.” The media benefits from reporting on disasters. Certain scientists benefit from extending the pandemic for both funding and fame. Scientists who have opposing views are no longer on mainstream media in the interest of preventing “vaccine hesistancy” and some have been ostracized by their peers. Alternative viewpoints of experts have been effectively stiffled.
- Cognitive dissonance. People don’t really change their minds and get cemented in the things that they learn. This happens all the time in physics. Quantum mechanics was mystifying and difficult to accept when first proposed. However, the next generation of physicists are taught it in first year university as completely obvious.
- Confirmation bias. Usually, people have made up their minds and look for data to confirm it. 107And all viewpoints can find facts that confirm their choice in this document. You are welcome in advance.
- Contrast-bias / Anchoring. Most media reports do not have comparisons with absolute numbers. The latest CDC report states that unvaccinated people are 29x more likely to be hospitalized. This sounds horrific and terrible.108“Here the great experiment that Cialdini does in his class is he takes three buckets of water. One’s hot, one’s cold, and one’s room temperature. And he has the student stick his left hand in the hot water and his right hand in the cold water. Then he has them remove the hands and put them both in the room temperature bucket, and of course with both hands in the same bucket of water, one seems hot, and the other seems cold because the sensation apparatus of man is over-influenced by contrast. It has no absolute scale. It’s got a contrast scale in it.” However, looking more carefully at the data, which is done later, we will see that the 29x number comes from a 7-day rolling average of age adjusted hospitalizations. In contrast, if we look at the average hospitalization rate difference between vaccinated and unvaccinated in that particular data set, it is 2x. If we had a further breakdown of numbers by age group, we would probably see much different results. Other datasets show that that for under 50 years old, there is hardly any difference in hospitalizations between vaccinated and unvaccinated.
- Sunk cost. Humans have a tendency to continue on any course of action even though there may be a better action available. Any cost that has occurred and cannot be recovered by any means shouldn’t be a part of future decisions, but it is.
9.3. Israel Normalized Data For Severe Cases
Age: < 50 | Unvaccinated/100k | Full/100k | Partial/100k |
---|---|---|---|
Cases | 6163.8 | 3199.8 | 2907.4 |
Severe | 54.9 | 1.8 | 4.4 |
Deaths | ? | ? | ? |
% Hospitalized | 0.9 | 0.1 | 0.2 |
% Dead of hospitalized | ? | ? | ? |
Age: >= 50 | Unvaccinated/100k | Full/100k | Partial/100k |
---|---|---|---|
Cases | 8517.4 | 2499.4 | 2410.9 |
Severe | 1523.1 | 123.5 | 257.4 |
Deaths | ? | ? | ? |
% Hospitalized | 17.9 | 4.9 | 10.7 |
% Dead of hospitalized | ? | ? | ? |
9.4. Israel Raw Data
This data was obtained from https://datadashboard.health.gov.il/COVID-19/general and the headers translated.
age group | cases unvax/ per 100K | cases vax/100K | cases part vax/ 100k | severely unvax/100K | severe vax/100K | severe part vax/100K |
---|---|---|---|---|---|---|
“12-15” | 1230.8 | 135.2 | 884.5 | 0.3 | 0 | 0 |
“16-19” | 1248.2 | 568.6 | 507.8 | 0.9 | 0 | 0 |
“20-29” | 1119.1 | 848.4 | 580 | 5 | 0 | 4.4 |
“30-39” | 1355.4 | 857 | 526.7 | 10.1 | 0.5 | 0 |
“40-49” | 1210.3 | 790.6 | 408.4 | 38.6 | 1.3 | 0 |
“50-59” | 1246.4 | 660.5 | 478.1 | 60.7 | 4.4 | 5.4 |
“60-69” | 1039.8 | 404.9 | 455.5 | 131.6 | 11.2 | 36.4 |
“70-79” | 2008.9 | 331.7 | 353.7 | 331.4 | 15.9 | 84.2 |
“80-89” | 1677.1 | 460.2 | 452.8 | 396.6 | 44.9 | 75.5 |
“90+” | 2545.2 | 642.1 | 670.8 | 602.8 | 47.1 | 55.9 |
“age group” | cases unvax | cases vax | cases part vax | Severe unvax | Severe vax | Severe part vax |
---|---|---|---|---|---|---|
“12-15” | 4282 | 271 | 617 | 1 | 0 | 0 |
“16-19” | 1458 | 2470 | 164 | 1 | 0 | 0 |
“20-29” | 2904 | 8447 | 262 | 13 | 0 | 2 |
“30-39” | 2550 | 8334 | 192 | 19 | 5 | 0 |
“40-49” | 1692 | 7357 | 115 | 54 | 12 | 0 |
“50-59” | 1006 | 4958 | 89 | 49 | 33 | 1 |
“60-69” | 656 | 2703 | 50 | 83 | 75 | 4 |
“70-79” | 388 | 1544 | 21 | 64 | 74 | 5 |
“80-89” | 203 | 964 | 18 | 48 | 94 | 3 |
“90+” | 76 | 300 | 12 | 18 | 22 | 1 |